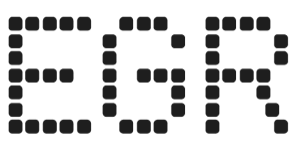
How xG stats benefit football punters more than operators
Simon Trim, strategic consultant for 10star, on why the use of complex data and customer behavioural analysis will bear fruit in the long run

There is a well-known saying that “the best prediction of future behaviour is past behaviour”. When applied to sporting contests at a high level it obviously makes sense – better teams and players beat worse ones. However, if it was always true then 5,000-1 underdogs like Leicester would never win the Premier League and betting on the outcome of sporting contests would be redundant.
In fact, the science and debate of trying to predict future outcomes of sporting events is becoming increasingly mainstream, largely driven by the ever-widening availability of data – think WinViz in cricket for example.
In soccer, the “expected goals” (xG) statistic is now ritually included in match summaries across the internet, Sky Sports and the BBC. Although not a predictive tool in itself, xG is a measure of shot quality and how likely that shot is to produce a goal when compared with many other shots taken from that position on the pitch – a shot with an xG of 0.4 being a 40% chance of scoring for example. For a low scoring sport like soccer, when the score presents only limited information on what happened in the game itself, statistics like this potentially offer real insight into whether that outcome was “fair” and therefore provide a better basis for predicting what future outcomes involving those teams might be, both for the operators that offer odds and for the customers that take them.
A useful metric for sure, but expected goals is also a great example of the problems associated with using “black box” data to predict an outcome or blindly following the output of a model, because xG values, and the models that produce them, are not equal.
Firstly, models are trained using historical data. The size of the data sample used, its quality and how it is interpreted all affect the outputted value.
Secondly, different xG models look at different levels of shot granularity. Simpler models look at whether the shot originated inside or outside the box, whereas more complex models look in greater detail at the angle of the shot or the method of attempt (header, shot) in assigning an xG value. Other models look at the passage of attacking play that preceded the shot, the position of the goalkeeper and so on. While in theory the more complex models should give a better predicted outcome, if the sample size isn’t high enough they also present a problem of “overfitting” the model to the specific data being sampled. There’s no one data set that leagues and data suppliers use, so we’re not always comparing like for like.
Data crunching
And herein lies one of the biggest pitfalls associated with using data in this way. While statistical outputs such as xG undoubtedly give us a better understanding of past behaviour, they don’t always give a better indication of what is coming next. This is especially so in looking at data without context – an early goal that a team defends successfully won’t translate into an analysis of their attacking performance through chances created.
However, for most sportsbook operators and suppliers, the use of more granular historical data, interpreting the context correctly and attempting to use elements such as xG as the basis for predictive tooling currently represents the frontier of sports algorithm development. Use of these “maths models” is necessary – and in some cases the models are reasonably advanced – but they are also by their very nature limited in terms of what they can predict and therefore how much they improve the quality of prices that bookmakers offer (and therefore the revenue that they generate).
So, what’s the solution for operators looking to improve the profitability of their operations and distinguish themselves from the competition?
The answer lies in the proper use of other forms of data that every sportsbook possesses – namely the size and type of liabilities being generated on their book and which customers generated them. It is also a form of data that is surprisingly (but almost universally) ignored by operators or suppliers.
There are various reasons for this ignorance that have evolved over the last 20 years or so. In short, they can be summarised as operators eschewing “traditional” odds compilation practices that lead to price differentiation (and the opportunity for profit maximisation) in favour of treating these prices like other forms of more basic content. There’s no need to invest into generating your own prices if you can buy them cheaply from suppliers that scrape them from “the market”. Instead, concentrate on land-grabbing customers by throwing money at mass-market advertising, free bets and promotions, then throw out the customers who make money out of your sub-standard pricing and you have the story of the betting industry since the dawn of online and in-play betting.
On current trajectory, it is also a story that doesn’t have a happy ending for most operators, as the landscape that led to the evolution of this model – low costs and the opportunity for previously untapped revenue – has long since eroded. Punitive data costs, improved social responsibility and affordability measures, expensive market-access deals, the high cost of technology and marketing plus the headwinds generated from the state of the wider economy has left many operators looking for an alternative way of generating higher revenue from the prices they offer their customers, without the in-house skill or the existing supply chain to provide the better odds they need.
So, what can operators do to close this gap? They need to look beyond sectoral norms and start to embrace the innovations that have underpinned exchange market-making and financial trading. All operators have rich seams of liability and customer data, but few are monetising them. By combining the best maths models with both real-time liability and customer behaviour data, they can start to generate better prices and therefore improve their bottom line.
The 25 years’ experience I have in sports betting has shown me that it’s only by using multiple forms of different data – statistical, financial and behavioural, all overlaid to solve the same problem – can sportsbooks maximise the chances of producing above average (or “alpha”) returns. Anyone utilising these automated techniques on all aspects of a sportsbook – from pre-match multiples, through same game parlays to in-play betting – suddenly accesses a differentiated business model that can thrive while less sophisticated competitors increasingly fail.
Simon Trim has over 25 years’ experience in the betting industry, including 15+ at board level. A driving force in bringing the sophistication of spread betting to power the growth in the B2B fixed-odds market, he is now strategic consultant to premium market-making and risk management service 10star. Recently launched by the same owners as Pinnacle, 10star is looking to utilise this heritage to modernise the sports betting industry by bringing some of the data innovations and risk management techniques of the financial markets to improve the bottom line for sportsbook operators.